Introduction
The martian atmosphere is mainly composed of CO2 (~ 95 %). The first spectral confirmation of CO2 cloud was acquired by Montmessin et al. [2007] using the 4.26 µm band of the ν3 fundamental asymmetric stretching mode of CO2 from OMEGA data onboard Mars Express.
Since then, numerous observational studies have constrained the climatology of CO2 cloud in the martian atmosphere [Määttänen et al., 2013]. There are two different types of clouds involving different formation processes: (i) those located in the troposphere at the winter polar region, and (ii) those located in the mesosphere at low and mid-latitudes during the martian year [Määttänen et al., 2013]. Microphysical processes of formation of theses clouds are still not fully understood. However, modeling studies revealed processes necessary for their formation: the requirement of waves that perturb the atmosphere leading to a temperature below the condensation of CO2 (transient planetary waves for tropospheric clouds [Kuroda et al., 2013], thermal tides [Gonzàlez-Galindo et al., 2011] and gravity waves for mesospheric clouds [Spiga et al., 2012]).
We use our microphysical model of CO2 cloud formation to investigate the occurrence of these CO2 cloud by coupling it with the Global Climate Model (GCM) of the Institut Pierre Simon Laplace (IPSL) [Forget et al., 1999]. We focus our efforts on the modeling of the tropospheric clouds during the winter in the polar regions.
Model description
The microphysical model of CO2 cloud formation of LATMOS includes nucleation on crystals on cloud condensation nuclei (CCN), condensation/sublimation, and sedimentation. Sources of CCN for CO2 are mainly dust particles, secondarily water ice particles. The particle size distribution is described with the moment method allowing to compute the effect of the microphysical processes on the average properties of the distribution. This method is the same as used for water ice clouds microphysics in the MGCM-IPSL [Madeleine et al., 2014, Navarro et al., 2014]. For more details about the microphysical processes of CO2 clouds, we invite the reader to the work of Listowski et al. [2013, 2014].
The MGCM-IPSL is a finite difference model based on the primitive equations of meteorology in σ coordinates [Forget et al., 1999]. The horizontal resolution grid used is 64 x 48, corresponding to 5.6258° longitude by 3.758° latitude, respectively. The top of the atmosphere was extended to ~ 120 km to describe well the processes in the mesosphere. The atmosphere is divided into 32 vertical layers from the surface to the top of the atmosphere. At each call of physical processes (every 15 minutes), the microphysics of CO2 cloud formation is called 50 times leading to a time resolution of 18 seconds to resolve the very rapid microphysical processes. We have used a dust scenario from the MY29.
Results
We present our first results on 3D modeling CO2 clouds in the Martian atmosphere. Figure 1 shows the zonal mean density column of atmospheric CO2 ice as simulated by the model during a Martian year. The maximum columns of CO2 clouds are found in winter polar regions. In the northern polar region, CO2 ice clouds form from around Ls = 220° at highest northern latitude, reaching the latitude of 50°N around Ls = 270°, and disappear slightly before the northern spring equinox at Ls = 350°. In the southern polar region, CO2 clouds are formed from around Ls = 0° at highest southern latitude, reaching the latitude of 54°S around Ls = 130°, and disappear at Ls = 190°.
This result is quite consistent with MCS for MY29 observation which showed CO2 ice cloud up to 68°N (Fig. 1a from Kuroda et al. [2013]) during the period Ls = 255°-285°. Note that their observational data came from MRO-MCS Derived Data Version 2 [Kleinböhl et al., 2009], where the dust profiles retrieved in winter polar regions are likely to be caused by CO2 ice clouds [McCleese et al., 2010]. Our CO2 ice clouds simulated are also qualitatively consistent with those observed from the Mars Orbiter Laser Altimeter (MOLA) (see Figure 2). The temporal distribution of simulated clouds in winter polar regions are in good agreement with MOLA observations.
Figure 3 and 4 show the zonal mean CO2 mass mixing ratio in both winter polar regions: between 60°-90°N averaged in time between Ls = 180°-360°, and between 60°-90°S averaged in time between Ls = 0°-180°, respectively. The density of CO2 ice clouds simulated are in agreement with the distribution of winter polar clouds observed by MOLA during the same Ls period (Fig. 8 from Neumann et al. [2003]). The thickness of CO2 saturation layer observed by MCS during the MY29 is around 8 km in the northern polar region between Ls = 180°-360° [Hu et al., 2012], lower than our CO2 ice cloud extension in altitude reaching around 20 km altitude. But the thickness of CO2 saturation layer in the southern polar region observed by MCS for the same year is around 15 km, nearly equal to our top of CO2 ice cloud below 15 km altitude.

Figure 1: Zonal mean density column of CO2 ice during one Martian year.
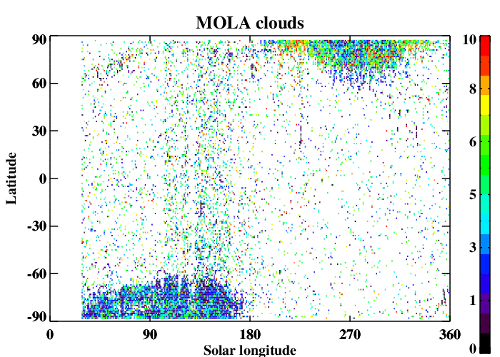
Figure 2: Cloud top altitude (km) from MOLA observations binned in 1°x1° latitude-solar longitude grid.

Figure 3: Zonal mean CO2 mass mixing ratio in the northern polar region as a function of altitude, averaged between Ls = 180° to 360°.

Figure 4: Same as Fig. 3, except for the south pole during Ls = 0° to 180°.
Conclusion
The microphysical module for CO2 clouds of LATMOS coupled with the Martian Global Climate Model of the Institut Pierre Simon Laplace has reproduced qualitatively the CO2 ice clouds in winter polar regions in the first full-year simulations. Further analysis needs to be done to complete the study of these CO2 ice clouds and compare more quantitatively to the observational data. We will also study more in detail the formation of mesospheric clouds in the martian atmosphere in the near future.
Acknowledgments
We thank our funders, the Agence National de la Recherche (project MECCOM, ANR-18-CE31-0013), the Laboratoire d'Excellence ESEP, and the French space agency CNES.