Introduction
Legacy methods which provide us with quantitative information on asteroid composition are based on spectral unmixing or specific spectral parameters (band depths, band areas, positions of band minima). These methods are sensitive to quality of input data and our apriori knowledge about the asteroid. We introduce a new approach based on artificial neural networks, which allows us to derive modal and chemical compositions of olivine-pyroxene-rich asteroids with precision better than 10 percentage points.
Data
We used measured reflectance spectra of olivine and pyroxene from the RELAB and C-tape databases. We selected spectra which were measured at least from 450 nm to 2450 nm with a resolution of 15 nm or better. Eventually, we interpolated these spectra to a wavelength grid with 5-nm spacing, denoised them using a convolutional filter, and normalised them at 550 nm. In total, we collected 510 reflectance spectra (100 olivine, 102 orthopyroxene, 108 clinopyroxene, 137 laboratory olivine-pyroxene mixtures, and 63 meteorites). For each spectrum, we have information about the sample modal abundances (in volume percent) and chemical composition (represented by end-members of individual mineral).
Model
An artificial neural network is a multi-parametric empirical model. Free parameters of the model are set (trained) according to input and output data. The neural networks are formed of layers of neurons. The basic layers are the input layer, the hidden layers, and the output layer. The layers are sequentially non-linearly connected. The non-linearity makes the neural-network model flexible enough to solve various tasks.
We trained a neural network for determining mineral modal abundances and mineral chemical compositions of olivine, orthopyroxene, and clinopyroxene, which are the major constituents of S-complex-like meteorites. The implemented neural network is made of an input layer, two convolutional hidden layers, and an output layer. The input layer inputs reflectance values at given wavelengths. The reflectance values are propagated through the model and result in the mineral modal abundances in volume percent and mineral chemical compositions represented by the mineral end-members.
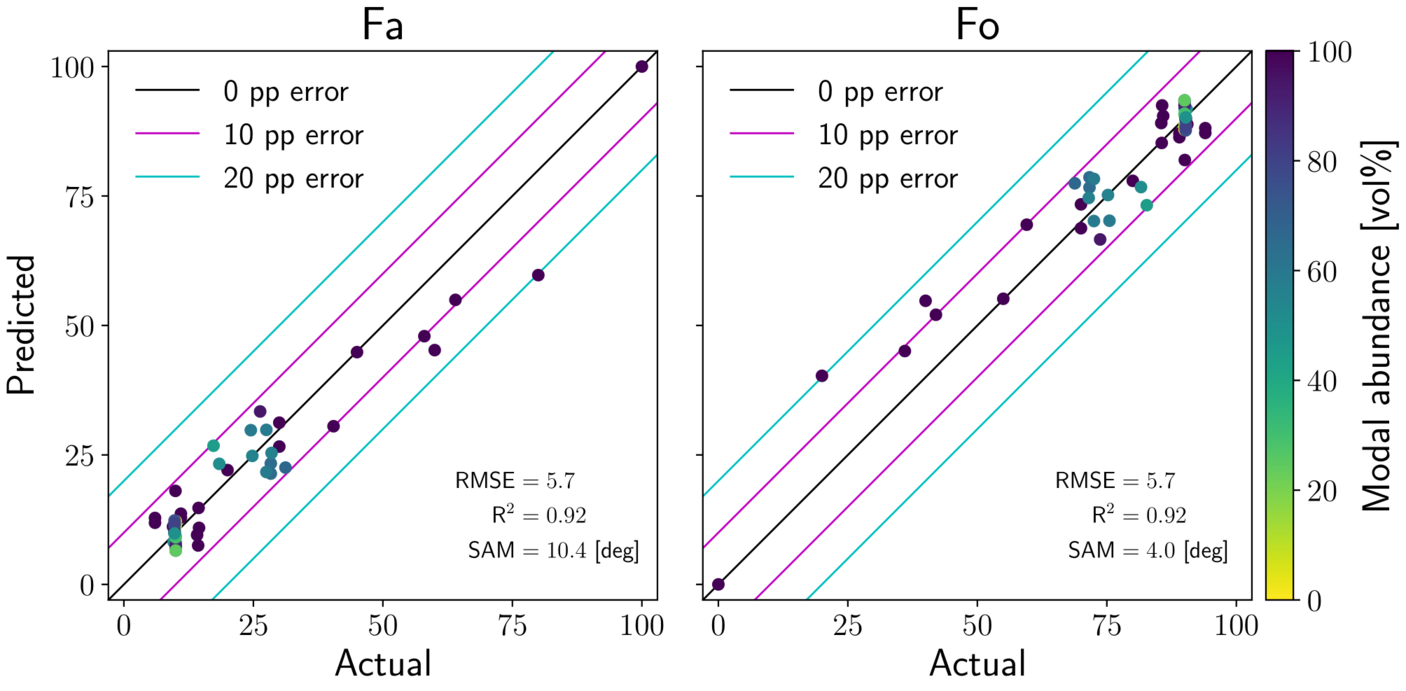
Results
We evaluated accuracy of the trained model on different set of olivine, pyroxene, and olivine-pyroxene-mixture spectra. We found that modal abundances can be estimated with RMSE better than 10 percentage points, and chemistry of olivine and orthopyroxene with RMSE of about 5.7 percentage points. RMSE of clinopyroxene chemistry is about 11 percentage points. The results for olivine chemistry is shown in Fig.1.
We applied the trained neural network on olivine-pyroxene-rich asteroid spectra (DeMeo et al., 2009; Binzel et al., 2019). We found a good agreement between S-type and Q-type asteroids and ordinary chondrites. Additionally, the model predicted that V-type asteroids are made of almost pure pyroxene, and A-type asteroids of almost pure olivine. The model revealed a systematic shift in olivine fraction between S-type and Q-type asteroids.
Discussion
We compared the neural-network predictions with the legacy band-area / band-centre-based methods (Cloutis et al., 1986; Gaffey et al. 2002; Reddy et al. 2015) and found that our predictions on modal abundances and chemistry are closer to the actual values and the validity region of our model is larger.
When analysing S-complex asteroid spectra, we observe apparent systematic depletion of olivine in S-type asteroids compared to Q-type asteroids, which have olivine abundance similar to those of ordinary chondrites. We hypothesise that this can be an effect of space weathering rather than compositional trend. Olivine undergoes space weathering changes in shorter timescale compared to pyroxene. Therefore, the space-weathering-attenuated absorption bands of olivine naturally results in relatively lower predicted olivine abundance compared to pyroxene.
We tested the hypothesis using (1) principal-component-based classification with determined space-weathering direction (Binzel et al., 2019), and using Chelyabinsk meteorite with (2) laboratory-induced space weathering and (3) its mixtures with spectrally featureless dark impact-melted or shock-darkened phases. The overall silicate mineralogical composition of Chelyabinsk meteorite in cases (2) and (3) remained consistent. In the PCA graph (1) in Fig. 2 and in weathered Chelyabinsk (2), we observed apparent depletion of olivine with increasing weathering, while the olivine abundance remained constant in the mixtures with darkened material (3). Even a large (50% and more) portion of spectrally neutral phase did not change predictions made by our model significantly.
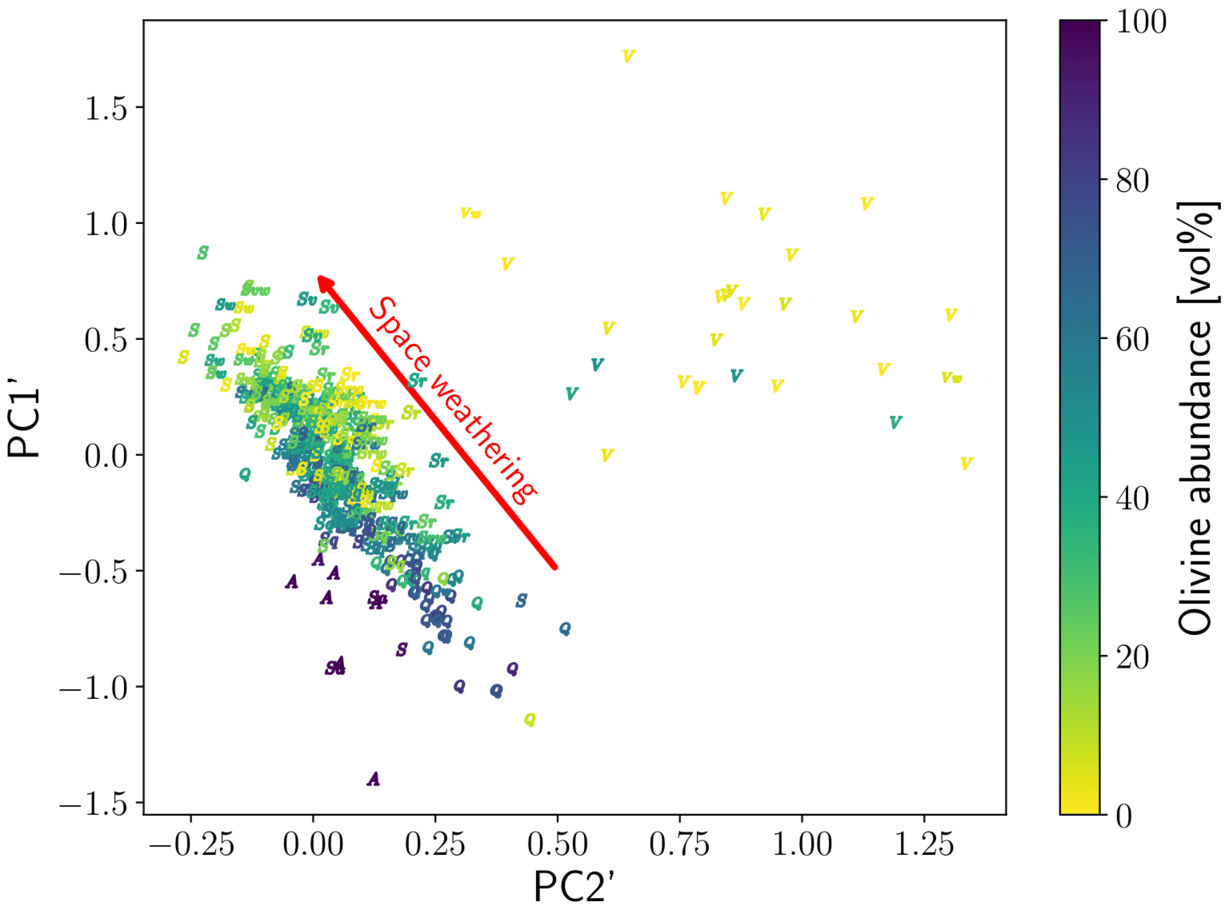
Conclusions
These results show that our model is sensitive to relative changes in strength of individual mineral absorptions, while at the same time is insensitive to wavelength-independent spectral attenuation. On weathered asteroids, the model is capable of finding locations which are fresher or more weathered if slowly varying modal abundance is assumed
References
Binzel, R. P., DeMeo, F. E., Turtelboom, E. V., et al. 2019, Icarus, 324, 41
Cloutis, E. A., Gaffey, M. J., Jackowski, T. L., & Reed, K. L. 1986, J. Geophys. Res., 91, 11, 641
DeMeo, F. E., Binzel, R. P., Slivan, S. M., & Bus, S. J. 2009, Icarus, 202, 160
Gaffey, M. J., Cloutis, E. A., Kelley, M. S., & Reed, K. L. 2002, in Asteroids III (University of Arizona Press, Tucson), 183–204
Reddy, V., Dunn, T. L., Thomas, C. A., Moskovitz, N. A., & Burbine, T. H. 2015, in Asteroids IV (University of Arizona Press, Tucson), 43–63